The Profound Impact of Machine Learning in Business
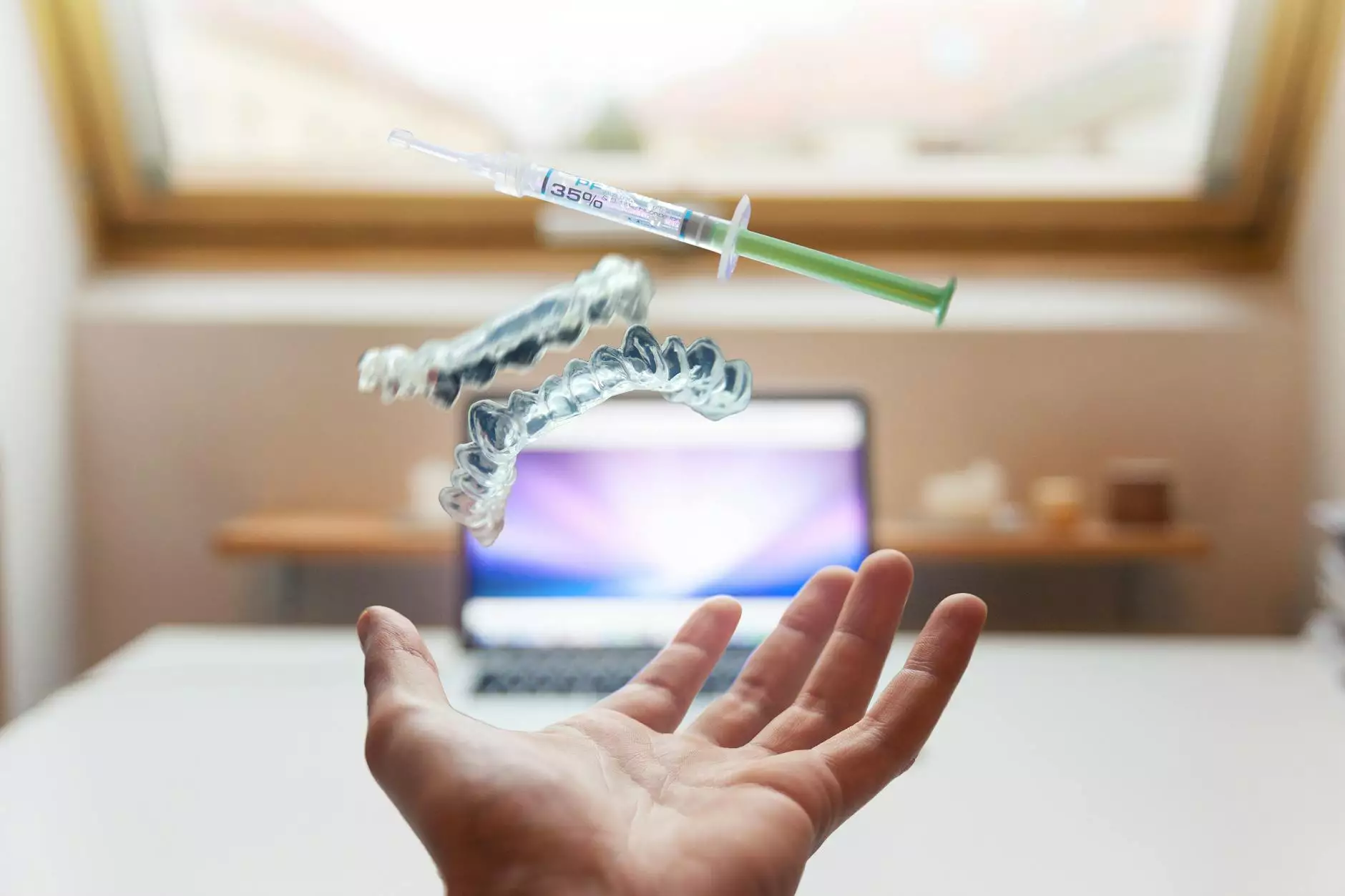
In today's rapidly evolving digital landscape, machine learning has emerged as a critical component of modern business strategies. It acts as a force multiplier, enabling businesses to analyze vast datasets and extract valuable insights that were previously unattainable. This article delves into the myriad ways machine learning is reshaping the business landscape through detailed analyses, real-world applications, and industry-specific case studies.
Understanding Machine Learning in a Business Context
Machine Learning (ML) is a subset of artificial intelligence (AI) that focuses on building systems that can learn from and make decisions based on data. In a business context, ML helps to automate processes, enhance customer experiences, and uncover patterns within massive datasets.
Key Concepts of Machine Learning
- Supervised Learning: Algorithms are trained using labeled datasets to predict outcomes.
- Unsupervised Learning: Algorithms explore unlabeled data to identify hidden structures without predetermined labels.
- Reinforcement Learning: Algorithms learn by interacting with an environment to maximize cumulative rewards.
The Business Applications of Machine Learning
There is a wide array of applications for machine learning articles that can inspire your business to harness this technology effectively. Here we explore some notable examples:
1. Enhancing Customer Experience
One of the most profound impacts of machine learning is its ability to transform customer interactions. Businesses can utilize ML algorithms to analyze customer data, predict preferences, and offer personalized recommendations.
Case Study: Netflix
Netflix utilizes machine learning algorithms to analyze viewer habits and preferences. By examining viewing history and user ratings, they can provide personalized recommendations. This has significantly increased user engagement and retention rates, showcasing how ML can enhance customer satisfaction.
2. Automating Processes
Automation through machine learning alleviates repetitive tasks, allowing employees to focus on more creative and strategic work. Tasks such as data entry, customer service inquiries, and inventory management can all be optimized using ML technologies.
Case Study: Amazon
Amazon employs machine learning to optimize its supply chain management. Through sophisticated algorithms, they can predict demand and manage inventory efficiently, thus reducing costs and ensuring product availability.
3. Predictive Analytics for Decision Making
Machine learning empowers businesses to leverage predictive analytics to forecast trends and make data-driven decisions. By modeling historical data, companies can anticipate market movements and adjust their strategies accordingly.
Case Study: Target
Target has successfully employed predictive analytics to identify customer trends and purchasing behaviors. Their ability to predict which products will be popular in the coming months has given them a competitive edge in retail.
Challenges in Implementing Machine Learning
While the benefits are evident, businesses must navigate several challenges when implementing machine learning solutions:
- Data Quality: High-quality data is essential for effective machine learning models. Poor data can lead to inaccurate predictions.
- Resource Allocation: Implementing machine learning requires significant investment in technology and skilled personnel.
- Change Management: Organizations must adapt to new technologies, which may require cultural shifts and training.
The Future of Machine Learning in Business
The future of machine learning in business looks promising, with continuous advancements driving innovation. Here are a few trends that are likely to shape the future landscape:
1. Increased Personalization
As machine learning technologies evolve, businesses will become increasingly adept at personalizing customer experiences. By analyzing behavioral data in real-time, companies can tailor their offerings to meet individual customer needs.
2. Integration with IoT
The convergence of machine learning and the Internet of Things (IoT) will further enhance operational efficiencies. Smart devices will continuously generate data, which machine learning algorithms can analyze to improve decision-making and automate responses.
3. Ethical Considerations
As machine learning becomes more prevalent, businesses will need to address ethical concerns surrounding data privacy and algorithmic bias. Establishing frameworks to manage these issues will be crucial to maintaining trust with consumers.
Conclusion
Machine learning is no longer just a technology for tech giants; it’s an integral part of the business landscape that any organization can leverage. By understanding the principles, applications, challenges, and future prospects of machine learning, businesses can position themselves as leaders in their respective markets. Effective use of machine learning articles can provide insights that drive innovation, improve operational efficiencies, and enhance customer experiences.
To stay competitive, businesses must invest in machine learning technologies and cultivate a culture of data-driven decision-making. Those who embrace this technology will undoubtedly unlock a wealth of opportunities in the ever-evolving world of business.